From Clicks to Cash: Investigating the Financial Bias of Algorithm-Driven Pyramid Scheme Promotion
In this new digital age, select online platforms have emerged as influential gatekeepers of user’s attention. Among these, YouTube stands as a behemoth, captivating billions of viewers with its diverse range of videos. But YouTube’s influence doesn’t stop there; your very interests are controlled as videos are recommended to you based on massive sets of statistical analysis – all shrouded behind the label of the “algorithm.”
There is a remarkable lack of research into how this algorithm functions, but one thing is certain: it isn’t perfect. It’s far, far from it.
Because of its “proprietary” status, little research has been published about the YouTube algorithm, of which most investigates the way YouTube traps people in misinformation bubbles. What hasn’t been explored, though, is the financial implications of the YouTube algorithm on different demographics of viewers.
Although the algorithm might not know the specific number you filed on your tax return, AI models in general have been found to create extremely good proxies for demographic information when given biased datasets. This in turn creates a feedback loop where the algorithm perpetuates bias that is often more obvious and harmful than it was before. In the context of YouTube’s AI, then, if they have unwittingly created a proxy for viewers’ income, suggesting harmful videos based on that information could be recklessly endangering their user base.
Through an audit of their AI, this paper will investigate whether YouTube’s algorithm is more likely to recommend videos about financial scams to its poorer demographic of users.
Methods
To investigate the effects a user’s financial income may have on the algorithm’s recommendation of pyramid schemes, two user profiles were created and executed with pre-defined search queries. The “poor” profile focused mainly on how to acquire payday loans, where the “rich” profile focused on creating trust funds. In part two of the audit, each profile searched “do mlm’s make money,” “doterra,” and “how does network marketing work,” and the videos listed were recorded as well as the order they occurred and the presence of any sponsored posts.
Audit Preparation
The questions posed by Sui et al. in Fig. 1 of their study were all considered before the audit began. The viewer was selected as the main focus of the audit, and a focused sample was selected due to time constraints. Content of videos and popularity metrics, although noted in data collection, were not used analytically due to the complexity of incorporating them into such a small sample.
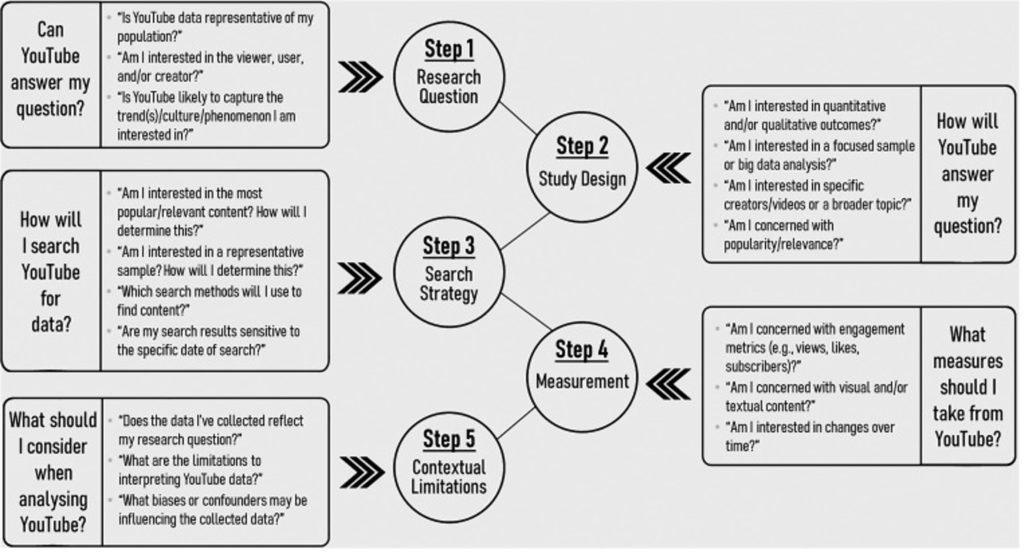
Sui, Sui, Rhodes – “What to watch: practical considerations and strategies for using YouTube for research”
In order to control the inputs of the algorithm enough to feasibly get a result from such limited data, it was necessary to meticulously mitigate the effects of my personal metadata on the audit. My own YouTube profile could not be used, and although the browser profile is separate it could be supplying third-party information to the YouTube algorithm. Before the audit began, many steps were taken to avoid this as completely as possible.
Using “Incognito” mode on both YouTube and Chrome was planned, but before beginning the audit it was decided that going Incognito on YouTube itself might change or limit the expression of the algorithm compared to the typical user. Thus, to continue to avoid the profiles influencing each other like YouTube Incognito might, a different computer was used for each.
Selecting proxy variables for financial income was certainly the most part of the preparation. Things that might represent closely a person’s wealth in the physical world – e.g. giant houses or frequent luxury trips – are often the subjects of videos that are appealing to a wide variety of viewers. Watching a video about a mega-mansion, per say, is not exactly a good indicator that one is interested in actually buying the property. Thus proxies had to be chosen with very specific target viewer demographics. Many options were considered, some being technical content on navigating specific banks’ online applications, home ownership v.s. renting, and personal bankruptcy v.s. reasonable investing content. The bank proxy required a lot of research into the clientele of the banks themselves, and using credit card companies seemed to skew more towards representing only lower incomes or less financial security; there wasn’t a clear “rich person” equivalent. Home ownership again felt too broad in viewership, and investing content was too subject to individual philosophies.
Ultimately the two proxies chosen were getting payday loans and setting up trust funds / holding companies. The mixture of two proxies for each category helped to lower the risks of unforseen algorithmic connections by diversifying the “interests” of each profile, and each felt specific enough that viewers interested in actually getting a payday loan or actually setting up a trust fund were in a vast majority. They also seemed to have decent correlations to viewer income, with the “poor” profile perhaps having a stronger connection than its “rich” counterpart – an effect that was present in almost every proxy consideration and thus deemed unavoidable on this small scale.
The part two queries chosen were “do mlm’s make money,” “doterra,” and “how does network marketing work.” The first was likely the most unbiased as the searches later would have the previous part two searches as input, but due to the small size of the audit having backups was relevant.
Audit Performance
The audit was performed at the Marriott Library in Salt Lake City, Utah, a location that was selected mostly due to transportation limitations. In the future, two separate libraries might be selected, one in a lower income area and one in a higher income area to further provide the algorithm financial data.
Data collection started at 9:39 AM and continued without interruption until after 5:00 PM. Data for two profiles was gathered, one “rich” and one “poor”, and only minor changes to the plan were made. Ads were not recorded as they were easy to miss, and for the same reason all ads were played in their entirety to remain consistent across the board. The volume was turned off on the computer itself and not the browser, and the captions were played in English for every video, varying only in how quickly they were turned on. In part two the “doterra” search had less videos recorded as the subsequent videos were largely unrelated.
Results
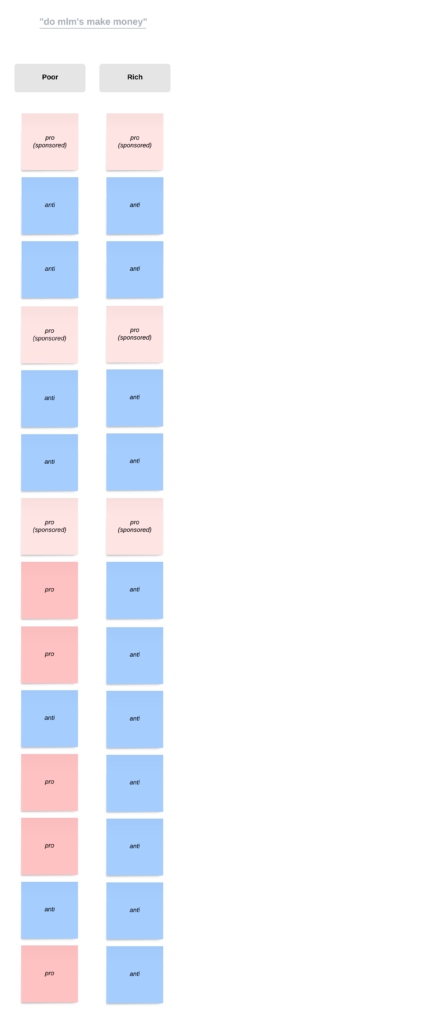
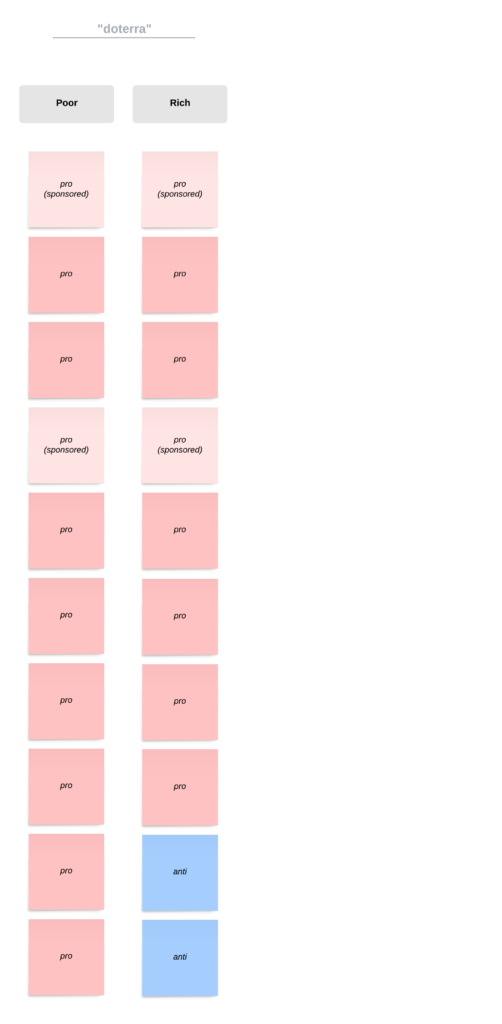
There were differences in algorithm output across profiles, and the “poor” profile saw more pro-mlm videos than the “rich” profile, as well as anti-mlm videos showing up earlier in the “rich” profile’s recommended videos. Especially at the beginning of the list, though, multiple videos were the exact same across profiles. This suggests either that the YouTube algorithm holds a very strong preference for those videos regardless of the viewer’s meta-data profile, or that the amount of videos each profile watched wasn’t significant enough to affect the top few recommendations. Given the small size of this audit, the latter is more likely. The third query, previously asserted to be the least accurate display of the algorithm’s disposition, recommended the exact same video types in the exact same order. While this was not expected, it makes sense given the proportionality of the part two searches versus the small number of videos watched.
Another unexpected phenomenon appeared in the nature of ads; although they weren’t specifically recorded, the difference in ads between the two profiles could not have been more obvious. The “poor” profile almost immediately began to display extremely long, clickbaity and scammy ads. Most ranged from 4 to 6 minutes, with some even being 10 minutes long. As an example, one ad that played multiple times was of a man opening package after package full of $100 bills. The man would fan them out, almost preening for the camera, enticing the viewer to click on their “opportunity.”
The “rich” profile was shown ads for things like Saint Jude’s hospital.
Discussion
While the dataset for this audit is much too small to fully understand the magnitude of this algorithmic bias, the trend displayed in even these short outputs is alarming. While recruitment-based MLM’s and product-based pyramid schemes are financially dangerous to anyone involved, it seems clear that a $500 loss could be devastating to someone already desperately looking into payday loans.
Especially in MLM companies like doTERRA – who require purchase of inventory to make commission – not only are the members highly unlikely to make any income, they could incur massive losses if their product doesn’t sell. These companies, as was reflected in the video content recommended, promote themselves as legitimate or even highly lucrative business opportunities. This idea should not be further promoted by YouTube, especially to those people who stand to lose the most.
Future Research
While this audit performed well as a proof-of-concept, future research into this algorithmic bias would likely require hundreds or thousands of profiles that contain much larger amounts of metadata. Actual YouTube profiles would likely need to be made for each, and the number of videos they watched increased dramatically. But because even this small scale audit took upwards of 9 hours – with constant babysitting – just to perform, a larger scale would prove overwhelming. Changes to the audit methods would be necessary.
A sock-puppet model would likely prove more useful, where bots are pre-programmed with a series of queries and videos to watch, allowing for drastic improvements in the feasible scale. While this may engage a significant amount of computer power, it massively increases the “inputs” in each profile, unrestricted by the capacity of human attention. This also creates more control over audit mechanics, as the bots’ programming would be simple and skipping ads could become standardized. In the same way, the captions, length between videos, and web engagement could be consistently implemented, as well as the ability to record more data about each session. A VPN could also be employed to include geographic proxies for each profile.
If individual YouTube profiles were created, the ability to comment, like, and subscribe would also become available. This promotes the profile from being a “viewer” to a “user,” again massively increasing the number of inputs each profile is able to feasibly give.
When entering part two of the audit, more consideration should be given to the query used. Ideally it should be one that, for a typical user, provides varied results. It can be seen in the “doTERRA” search of this proof-of-concept that some queries may return mostly skewed or unrelated recommendations when the topic is too specific, but leaning too far into non-specification is likely to produce a similar result.
It should also be considered whether this type of audit is even legal.
Cultural Relevance
As Karen Hao put it in the MIT Review, “Algorithms now decide which children enter foster care, which patients receive medical care, which families get access to stable housing. Those of us with means can pass our lives unaware of any of this. But for low-income individuals, the rapid growth and adoption of automated decision-making systems has created a hidden web of interlocking traps.” While the implementation of such algorithms is perhaps inevitable, placing such blind faith in systems obscured by the words “trade secret” is allowing us to perpetuate the same discrimination as before but without the means to readily discover its existence.
The YouTube algorithm may seem harmless; all it does is connect metadata to videos you may find interesting. But assuming it can operate in a manner absent of human bias or systemic harm is an objective misunderstanding of what AI even is.
“The [AI] hype becomes problematic when it leads to what I call “data fundamentalism,” the notion that correlation always indicates causation, and that massive data sets and predictive analytics always reflect objective truth. Former Wired editor-in-chief Chris Anderson embraced this idea in his comment, “with enough data, the numbers speak for themselves.” But can big data really deliver on that promise? Can numbers actually speak for themselves?”
– Kate Crawford, Harvard Business review
As is supported by this audit and by a large body of research, our algorithms are only as good as the data we put into them. If we give an AI biased data, we create a decision-making machine that will operate in a biased way; garbage in gives garbage out.
In the context of this audit, connecting low income viewers to companies designed to prey upon them is a significant risk to their financial well-being. In a study cited by the FTC, it was found that – based on tax filings – only .4 % of people in product-based pyramid schemes and recruitment-based MLM’s made any money at all. In direct contrast, those gambling on roulette at Caeser’s Palace were over 7x more likely to see a profit. It’s not a stretch to see why promoting videos that promise massive rewards is unethical for the 99.6% that will lose money after they click.
Perhaps the use of AI is inevitable, but its progression should not come without regulation. We have the right to know what information these systems operate with, and when they’re found to perpetuate harm there should be a route to mitigate it. Especially in the MLM capital of the world, throwing the most vulnerable population into a pit of predatory scams is inexcusable.
Sources
Abul-Fottouh, D., Song, M. Y., & Gruzd, A. (2020). Examining algorithmic biases in YouTube’s recommendations of Vaccine videos. International Journal of Medical Informatics, 140, 104175. https://doi.org/10.1016/j.ijmedinf.2020.104175
This paper found that YouTube’s recommendation of videos related to vaccines displayed a homophily effect and thus a filter bubble effect; the recommendations of the algorithm were significantly influenced by the like-mindedness of each video. While viewers of the pro-vaccine videos may be less likely to be exposed to misinformation, this effect also means that viewers of the anti-vaccine content are less likely to be recommended videos from credible sources. Their findings support the feasibility of my audit and suggest that inducing the algorithm purely through watching videos is a valid method.
Google. (n.d.). Browse YouTube while incognito on mobile devices. Google. https://support.google.com/youtube/answer/9040743?hl=en
This is a support article on the effects of the YouTube Incognito function. It details that the user’s account activity (watch history, subscriptions, etc.) will not influence results when in Incognito mode. Essentially the experience is as if the user is not signed in to their account. This demonstrates that Incognito mode is a viable option for deinfluencing the effects of my personal internet footprint on the outcome of the audit.
Miller, T., Kando-Pineda, C., Heras, G. de las, Méndez, R., & Puig, A. (2022, November 25). Multi-level marketing businesses and Pyramid Schemes. Consumer Advice. https://consumer.ftc.gov/articles/multi-level-marketing-businesses-pyramid-schemes
This is consumer advice from the US Federal Trade Commission advising people on the nature of MLM’s and how they may be pyramid schemes. They assert that “most people who join legitimate MLM’s make little or no money,” as well as advising that these ventures can be especially damaging when the participant can’t easily afford the risk. This advice from the FTC serves to define an often predatory relationship between MLM’s and their distributors, especially when the individual has low fiscal risk tolerance.
Nilsson, A., Björk, J., & Bonander, C. (2022). Proxy variables and the generalizability of study results. American Journal of Epidemiology, 192(3), 448–454. https://doi.org/10.1093/aje/kwac200
This paper explores the complexity of using proxy variables that will later be used to generalize findings across a population. They provide types of proxies that can be used and their relationships to different outside influences, and statistical relations are also provided for extrapolating results to larger populations. I likely won’t be doing statistical analysis to this degree on my audit, but their paper was valuable in considering the type of relationship my proxy will have to the complicated considerations of the YouTube algorithm.
Rieder, Bernhard (2015). YouTube Data Tools (Version 1.42) [Software]. Available from https://ytdt.digitalmethods.net
This is a collection of software that allows the user to extract data from the YouTube platform. It works via the YouTube API v3, and is what Abul Fottouh et al. used to collect their data. This also isn’t as viable a tool for a smaller-scale audit like mine, but may be useful in collecting data on specific channels when I am analyzing my results.
Sui, W., Sui, A., & Rhodes, R. E. (2022). What to watch: Practical considerations and strategies for using YouTube for research. Digital Health , 8, 205520762211237. https://doi.org/10.1177/20552076221123707
This paper outlines study considerations when using YouTube for academic research. It addresses many different contexts and gives examples / approaches for each. The advice on bias (e.g. age, interaction) will be very valuable to consider when evaluating my results. In order to mitigate this, I will be closely considering my methodology using their Fig. 1 and suggested questions to consider.
Taylor, J. M. (n.d.). MLM Profit and loss rates vs. various income options. Federal Trade Commission.
This contains results from a study that was published by the FTC. It demonstrates the probability of earning money in a product-based pyramid scheme or recruitment-based MLM compared to other income earning strategies. Taylor shows that participants in these companies would be over 7x more likely to earn any money by gambling on roulette at Ceasar’s Palace. This further demonstrates the predatory nature of MLM’s especially when the participant doesn’t have a lot of money to “gamble.”
caption